Introduction to Explainable AI in Healthcare
Artificial Intelligence (AI) is rapidly transforming healthcare, from helping doctors analyze diagnostic images to assisting with personalized treatment plans. But as AI becomes more integrated into medical practices, healthcare professionals often face a big challenge: understanding the decisions that role of AI systems make. When AI functions as a “black box,” offering predictions without clear reasoning, it can leave doctors and other professionals uncertain about its reliability. This is where Explainable AI (XAI) comes in.

Explainable AI is designed to make AI decision-making transparent. For healthcare providers, XAI can explain why an AI system made a specific recommendation or diagnosis. This transparency is crucial for building trust in AI systems and ensuring patient safety. In this article, we’ll explore how XAI works, why it’s important in healthcare, and how it’s making a difference in the quality of patient care.
This transparency also extends to patient communication. When doctors can clearly explain the AI’s reasoning to their patients, it builds trust in AI-driven care. Additionally, XAI helps healthcare institutions meet regulatory requirements, as transparency is becoming increasingly critical in compliance with ethical and legal standards.
The Importance of Explainable AI for Healthcare Professionals
In healthcare, trust and accuracy are everything. When healthcare professionals use AI for diagnostics, treatment recommendations, or patient monitoring, they need to understand why an AI system has arrived at a specific conclusion. Unlike other fields, healthcare decisions impact human lives directly, so an unexplained AI decision can’t be taken lightly.
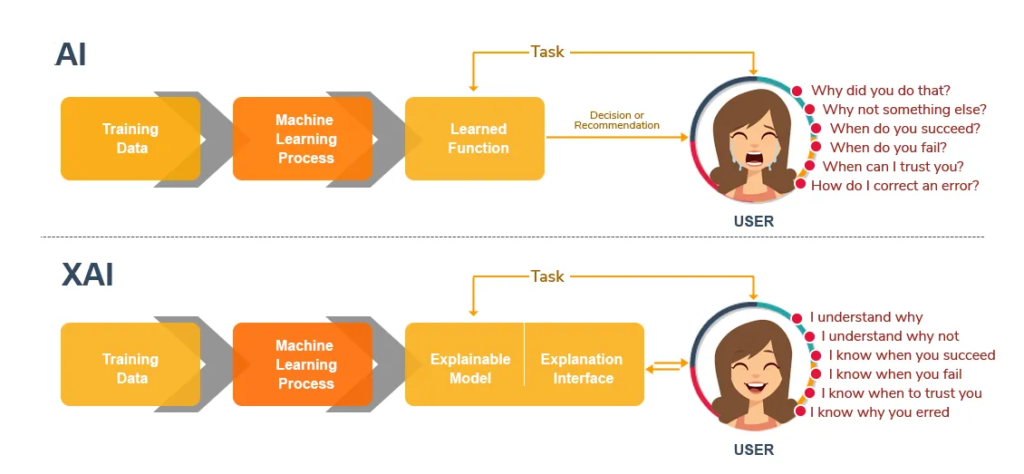
Explainable AI addresses this by providing healthcare professionals with insights into the factors and patterns behind its predictions. For instance, if an AI model flags a high risk of diabetes for a patient, XAI can clarify whether that prediction was based on family history, recent blood sugar levels, or other factors. Knowing these details helps professionals make informed choices, enhances patient trust, and improves overall treatment accuracy.
In healthcare, every decision affects a patient’s life, so it’s crucial for healthcare providers to fully understand the reasoning behind AI-generated insights. Trust is the foundation of effective patient care, and Explainable AI fosters that trust by breaking down complex AI predictions into understandable components.
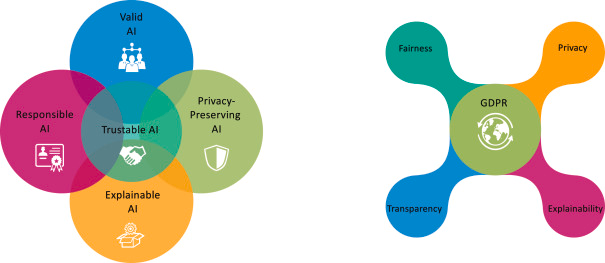
For example, if an AI system predicts a high risk of heart disease for a patient, XAI can clarify whether this prediction was based on factors like age, blood pressure, cholesterol levels, or lifestyle habits. By providing healthcare professionals with a clear understanding of how and why these predictions were made, XAI empowers them to make more confident, evidence-based decisions.
How Explainable AI Works
To make AI decisions more understandable, Explainable AI employs different techniques that help break down complex algorithms into easy-to-understand explanations. Here are some methods commonly used in healthcare:
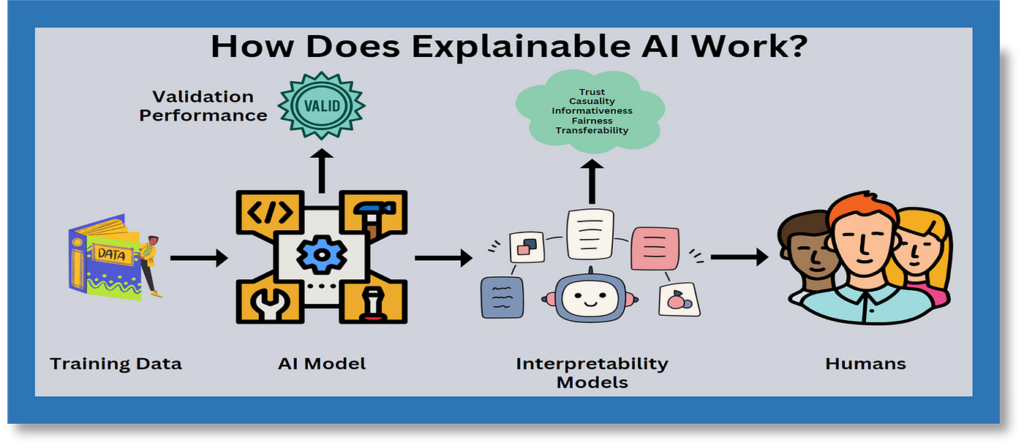
- Interpretable Models: Some AI models are inherently interpretable, meaning that their decision-making process is transparent from the start. Decision trees, for example, allow healthcare professionals to see exactly how each input (such as age, blood pressure, or lifestyle) contributes to the output (such as a diagnosis or risk assessment).
- Feature Importance Techniques: These methods highlight the specific factors (or “features”) that most heavily influenced the AI’s decision. For instance, a feature importance chart could show that factors like cholesterol levels and age were highly influential in predicting heart disease risk.
- LIME (Local Interpretable Model-Agnostic Explanations): LIME works by creating simple, interpretable models that approximate the complex AI model for specific predictions. For example, if an AI system predicts that a patient has a high risk of a certain condition, LIME can create an easy-to-understand summary showing the factors that led to this prediction.
- SHAP (SHapley Additive exPlanations): SHAP assigns each feature a “SHAP value,” which indicates its contribution to the AI’s prediction. In a healthcare setting, SHAP can help providers understand exactly how each patient’s risk factors influenced the AI’s output.
By using these techniques, Explainable AI helps make advanced AI models more transparent, ensuring healthcare professionals can trust and understand the AI’s conclusions.
Benefits of Explainable AI in Patient Care
Implementing XAI in healthcare provides many advantages that directly benefit patient care:
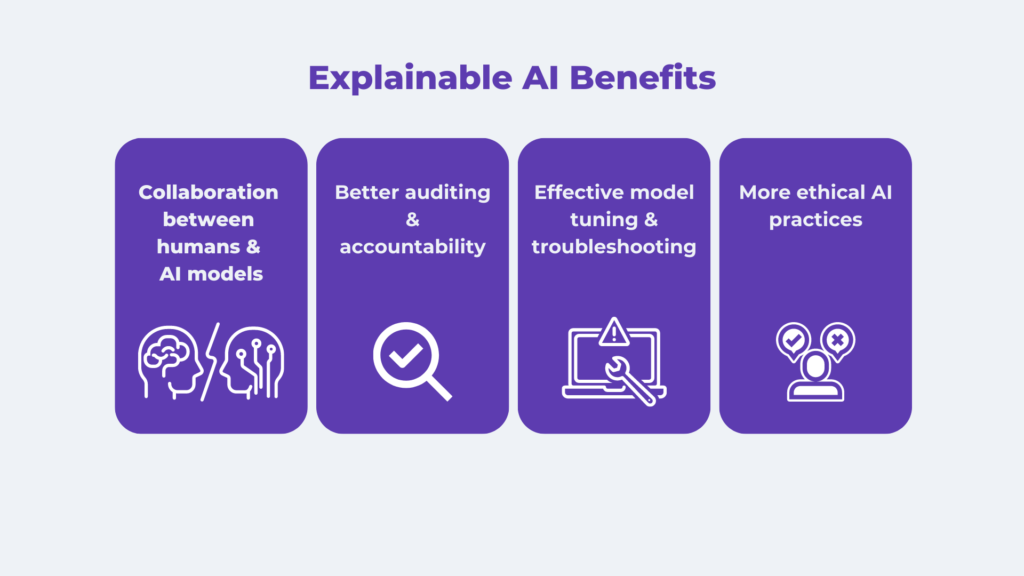
- Increased Trust in AI Systems: When healthcare providers understand the reasoning behind an AI’s prediction, they’re more likely to trust and rely on the technology. This confidence leads to better patient outcomes as professionals can confidently integrate AI insights into their practice.
- Better Patient Communication: Patients often have concerns about AI-driven decisions, especially when they’re related to health. Explainable AI enables doctors to explain AI-based recommendations in simple terms, easing patients’ worries and enhancing their trust.
- Enhanced Diagnostic Accuracy: With XAI, healthcare providers can validate AI-generated diagnoses by understanding the factors involved. This means they’re less likely to misdiagnose or overlook critical information, which ultimately leads to safer and more accurate treatment.
- Regulatory Compliance: Regulatory bodies increasingly expect transparency in AI applications, especially in healthcare. Explainable AI helps organizations meet these regulations by ensuring AI decisions are interpretable and justifiable.
- Continuous Learning and Improvement: XAI enables healthcare professionals to identify patterns or anomalies in patient care, leading to ongoing learning. By reviewing AI decisions, professionals can refine their diagnostic skills and improve their understanding of complex conditions.
- Building Trust and Reducing BiasTrust in AI is vital, especially in healthcare, where decisions directly affect patient lives. Explainable AI helps doctors understand how the AI system works, increasing trust in its recommendations. Additionally, XAI reduces bias by making the decision-making process visible, allowing healthcare providers to spot and address potential biases in AI predictions.
- Compliance with Ethical and Legal Standards:Explainable AI can help institutions meet legal requirements related to transparency and fairness, such as the GDPR’s “right to explanation” in Europe. By providing interpretable results, XAI supports ethical AI use and ensures that healthcare providers remain compliant with regulations.
Applications of Explainable AI in Healthcare
Let’s dive into some specific ways Explainable AI is already being used in healthcare settings:
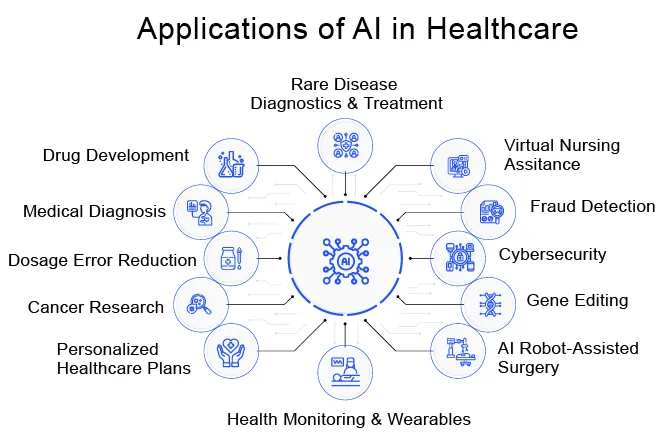
1. Diagnostic Imaging
In diagnostic imaging, AI assists doctors in detecting abnormalities in medical images, such as X-rays, MRIs, and CT scans. Explainable AI helps doctors understand which areas in the images triggered the AI’s findings. This can be crucial in detecting conditions early, like tumors, where accurate identification can significantly impact treatment outcomes.
2. Predictive Analytics in Patient Care
AI can predict health risks by analyzing patient data over time. For instance, it might predict the likelihood of a patient developing complications after surgery. With XAI, healthcare providers can understand which risk factors contribute most to these predictions, enabling them to take preventive actions or adjust treatment plans.
3. Treatment Recommendations
In some cases, AI systems recommend treatments based on past patient data and outcomes. Explainable AI helps healthcare providers understand why a certain treatment is suggested, allowing them to discuss and consider it alongside traditional treatment options.
4. Chronic Disease Management
AI systems used for managing chronic conditions, such as diabetes or heart disease, rely on data from patients’ health records, lifestyle, and monitoring devices. Explainable AI ensures that when an AI system suggests adjustments to a treatment plan, healthcare providers can see the data-driven reasons, helping them make better-informed decisions.
5. Early Disease Detection
Early detection of diseases like cancer can significantly improve patient outcomes. Explainable AI models analyze patient records and flag individuals who might be at high risk for certain conditions. XAI clarifies which factors contributed to the high-risk assessment, helping doctors conduct follow-up tests or screenings for these patients.
Popular Explainable AI Tools in Healthcare
Several Explainable AI tools are particularly useful in healthcare, each offering unique features for making AI-driven decisions more transparent:
1.LIME (Local Interpretable Model-Agnostic Explanations):
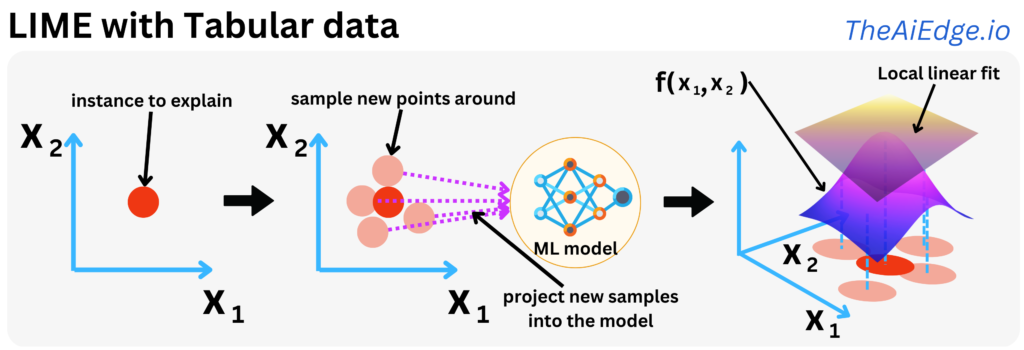
- Best suited for explaining individual predictions.
- Healthcare application: LIME can clarify why an AI system flagged a particular patient as high-risk, which helps providers take preventive actions.
2.SHAP (SHapley Additive exPlanations):
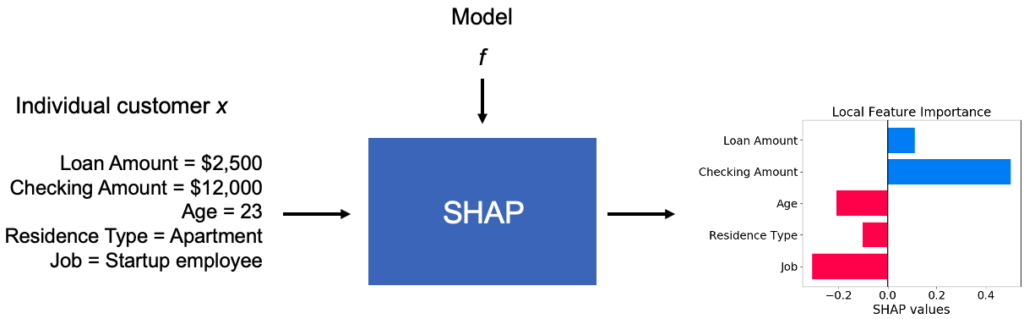
- Provides a detailed breakdown of how each factor contributed to a prediction.
- Healthcare application: SHAP values can help explain the risk factors for a heart disease prediction, making it easier for healthcare providers to discuss these risks with patients.
3.DeepSHAP:
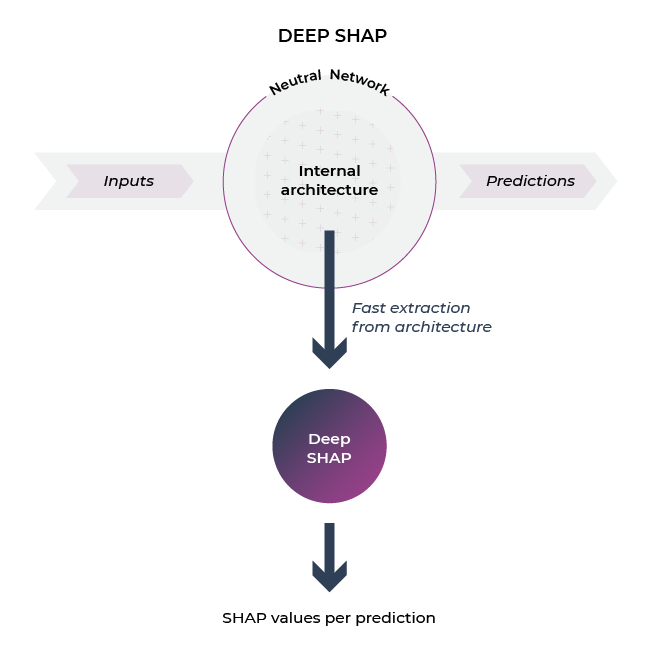
- A variation of SHAP that combines deep learning with explainable outputs.
- Healthcare application: Useful in analyzing complex data like genetic information, where traditional SHAP may not work as effectively.
4.IBM Watson OpenScale:
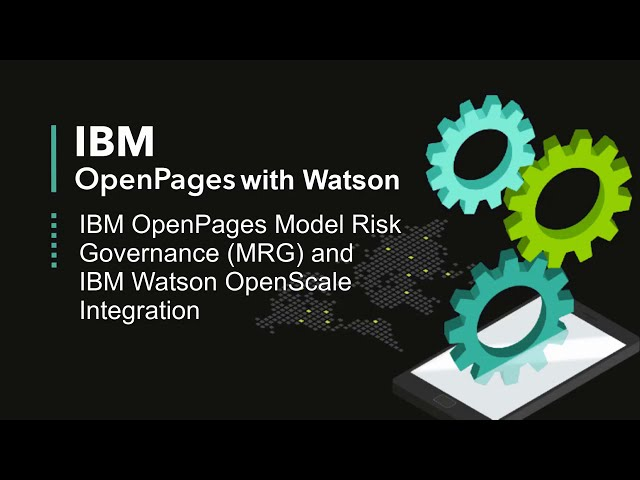
- IBM Watson’s platform for AI transparency and bias detection.
- Healthcare application: Used to track and interpret AI recommendations in critical settings like diagnostic labs or hospitals, allowing healthcare providers to understand and control the AI’s decision-making process.
5.Google’s What-If Tool The What-If Tool allows users to analyze how different variables affect AI predictions. In healthcare, it’s useful for exploring how changes in patient factors (e.g., BMI, blood pressure) alter risk predictions, enabling doctors to understand the AI’s sensitivity to different patient metrics.
These tools are gaining popularity because they enable healthcare professionals to understand AI decisions without needing a deep understanding of machine learning.
Case Studies and Real-World Examples of Explainable AI in Healthcare
Let’s look at a couple of real-world scenarios to see how Explainable AI can transform patient care:
Case Study 1: XAI in Cancer Detection
In a study conducted at a leading hospital, Explainable AI was integrated into their cancer diagnostics. The AI model helped radiologists identify potential tumors on MRI scans, highlighting regions of interest and explaining its reasoning. This led to faster diagnosis, enabling doctors to initiate treatment sooner and improving patient outcomes.
Case Study 2: AI in Diabetes Risk Prediction
A healthcare provider used Explainable AI to predict diabetes risk based on patient data. The AI flagged certain patients as high-risk due to lifestyle factors and genetic predispositions. Explainable AI provided a clear breakdown of how each factor influenced the prediction, which helped the doctors develop tailored lifestyle recommendations for these patients.
Challenges and Limitations of Explainable AI in Healthcare
While Explainable AI holds great potential, it also comes with challenges:
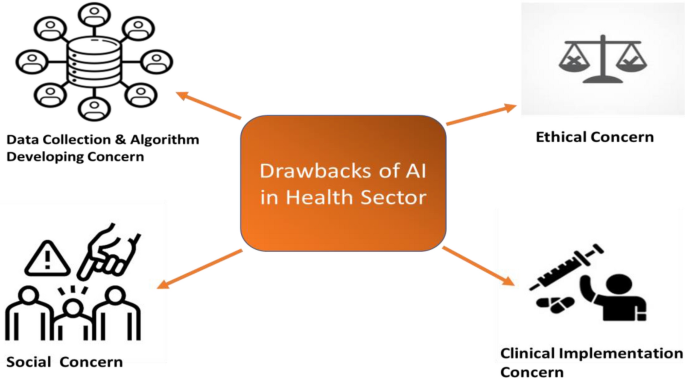
1. Complexity in Implementation
Many Explainable AI methods require technical expertise to implement and interpret correctly. Healthcare professionals may need additional training to understand how XAI works, which can be time-consuming.
2. Balancing Transparency and Privacy
Some AI explanations require detailed patient data, which raises concerns about privacy and data security. Healthcare providers must find a balance between transparency and protecting sensitive information.
3. Risk of Over-Reliance on AI
Explainable AI makes it easier for healthcare providers to trust AI, but there’s a risk of over-relying on these systems. Human judgment remains critical, especially in cases where AI may misinterpret unusual patient data.
4. Ethical Concerns
AI explanations might introduce biases,
Future of Explainable AI in Healthcare
As AI technology advances, the demand for transparency will continue to grow, particularly in healthcare. We can expect Explainable AI to evolve in the following ways:
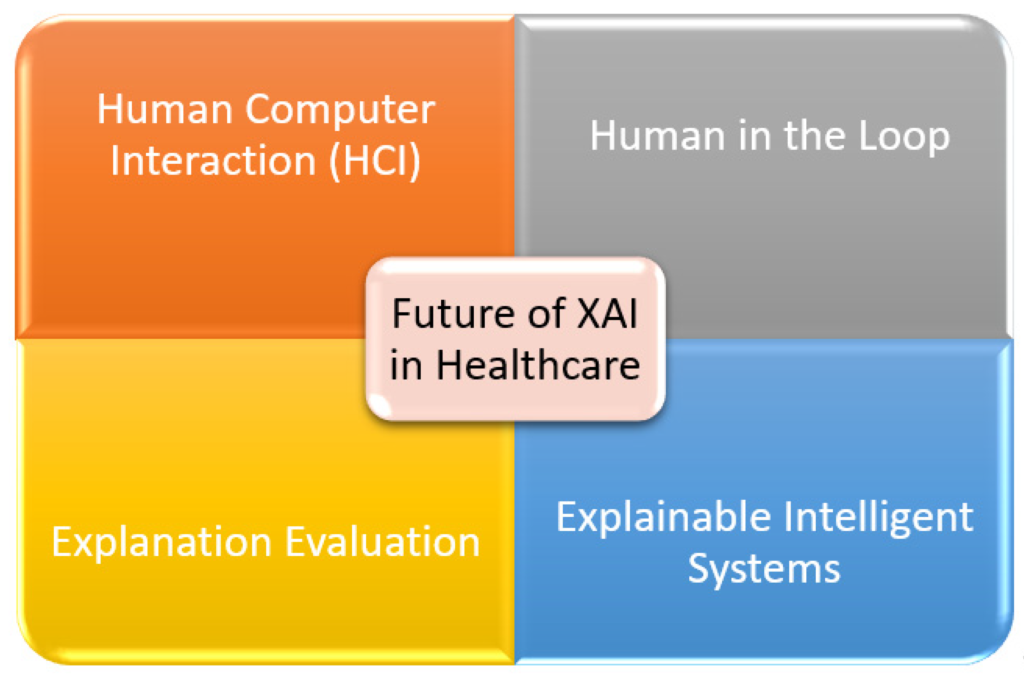
- Integration with Government Policies: More governments are likely to require explainable AI in healthcare to ensure patient safety and uphold ethical standards.
- Personalized Medicine: XAI could enable highly customized treatment plans by providing transparency in complex AI models that recommend specific therapies based on genetic profiles.
- Better Tools for Non-Technical Users: Future XAI tools will likely become easier for non-technical healthcare providers to use, expanding their accessibility and impact on patient care.
Conclusion
Explainable AI is not just a trend—it’s an essential technology for ensuring safe, trustworthy AI in healthcare. By making AI decisions transparent, healthcare professionals can make better-informed choices, communicate effectively with patients, and ultimately deliver better care. For healthcare providers who want to integrate AI into their practice responsibly, Explainable AI is a valuable tool for transforming patient care while maintaining the highest ethical standards.